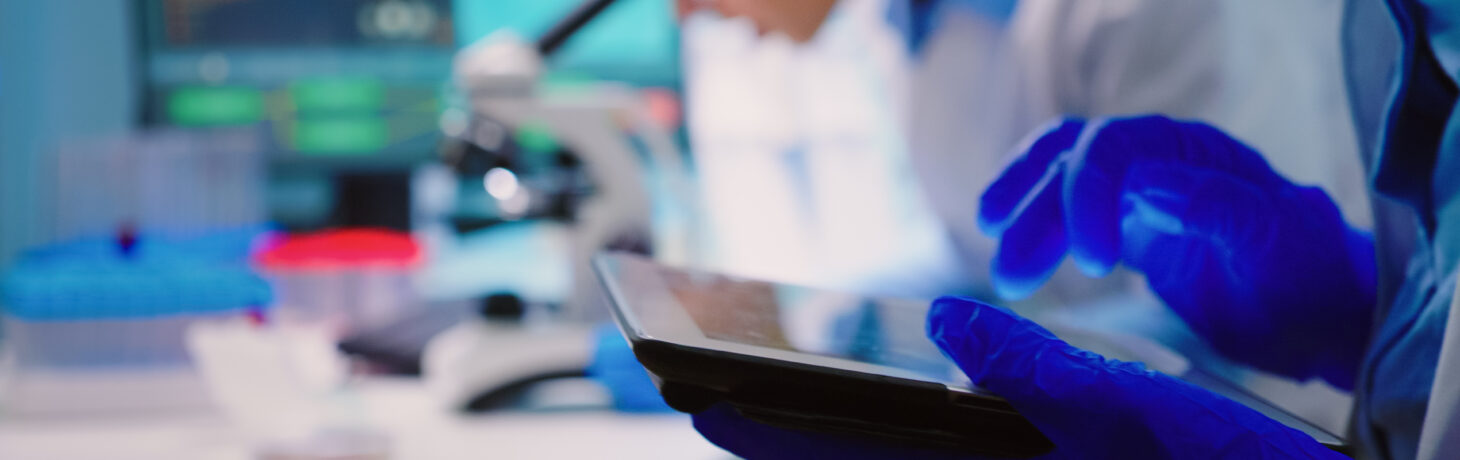
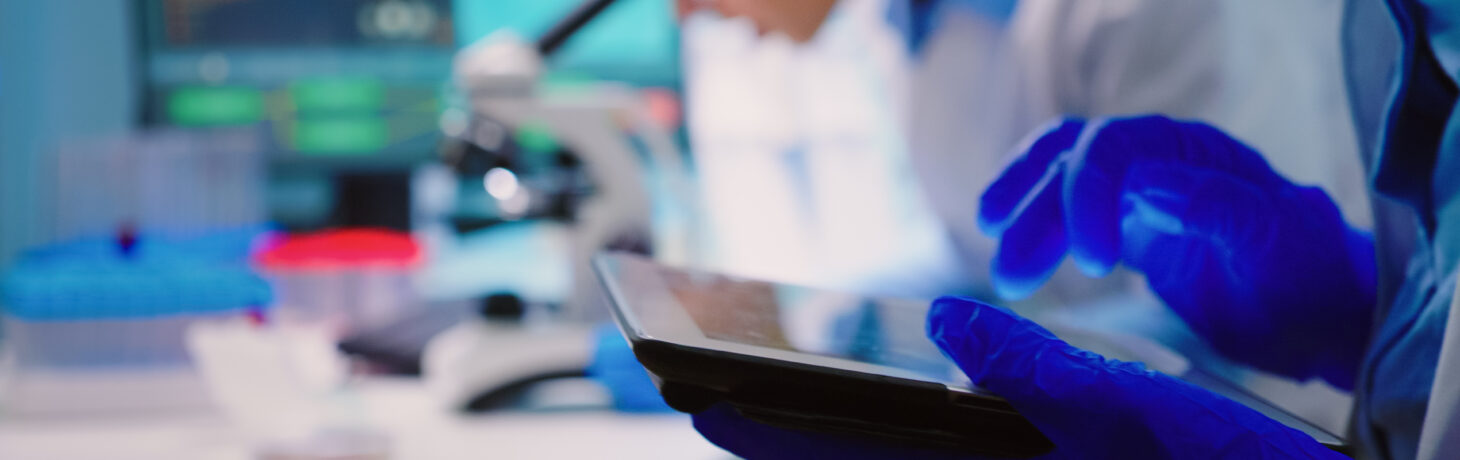
insights
Generative AI-Driven Clinical Trials: Are We There Yet?
This article is based on the session titled, “Generative AI-Driven Clinical Trials: Myth or Reality,” at the DIA 2024 Global Annual Meeting, in San Diego, June 2024. Many thanks to the presenters: Sharmin Nasrullah (Salesforce), Lichen Shen (Medidata), Aman Thukral (Abbvie), Lindsay Hughes (IQVIA), Jonathan Shough (Parexel), and Chunky Satija (Everest Group) for their valuable insights. This translation is the author’s rendering of their points and should not be taken as exact quotations.
As we integrate artificial intelligence (AI) into clinical trials, it is crucial to establish guiding principles to prevent harm. Often, there is a temptation to prioritize speed over sustainability. Meanwhile, reproducibility and auditability are essential regulatory expectations for our industry.
AI solutions must track information sources, and clinical trial processes should enable teams to build value from the vast data generated. Scaling efforts can be achieved through:
- Transcription: Converting languages, text to code, or explanations.
- Automation: Reviewing data, financial information, and creating forms.
- Generation: Producing visualizations, synthetic data, or compound structures.
Embracing AI Innovations
Currently, many users rely on click-based user interfaces (UI), but the future lies in conversational UIs (i.e., ChatGPT, chatbots, Alexa), and our approach must evolve to leverage AI advancements.
Clinical trials begin and end with data and documentation. Moving from hard-coded logic to large language model (LLM)-based workflows will revolutionize data integration, connecting sponsors, Contract Research Organizations (CROs), sites, and patients.
Simultaneously, we can apply personalization to patient engagement and glean insights into patients’ previous healthcare interactions, use systems and sites to foster better patient engagement, and use the data collection for real world data.
AI’s Role in Clinical Trials
AI’s potential in clinical trials is vast. Generative AI can simplify communication and translate complex data into layperson language, so why do we continue to burden the patient with complex data and scientific jargon in the clinical trial process? We can’t throw a lot of data into a system, retrieve the data, and think patients will understand the complexities of the research. But we can implement generative AI to simplify communication to provide better translation to patients in a way they can understand and comprehend. Using tools like ChatGPT to bring information down to the layperson language enables better interaction and a better overall experience for the patient, while still showcasing to the clinical trial sponsor areas that need more simplification.
Measuring AI Effectiveness
Key performance indicators (KPIs) for AI in clinical trials include patient recruitment, site quality, site selection, and predicting protocol deviations. Implementing generative AI in literature reviews is one example of how we are integrating AI into our business practices. We need to do a better job of understanding and owning data quality across sponsor organizations. Is there a data governance plan? Is the data consistent between users who input and those who read outputs? These are all human-led ways we can make AI work.
At Parexel, compliance with data quality is an annual performance review metric, measuring on-the-job action instead of training completion. Not all data is worthy of being input, so it’s critical to consider how you will use the data, how you will measure the data, and what privacy standards will be upheld. These questions require the strategy of humans to dissect and determine how we bring AI into our clinical trials.
Challenges and Considerations
Adopting generative AI at scale requires a mindful approach. Data quality and bias must be addressed, and the regulatory framework requires alignment. Data governance and inherent biases in AI, particularly towards a white male perspective, must be addressed. Training AI models and scrutinizing who creates these platforms are essential steps in ensuring ethical and inclusive AI integration in clinical trials.
Clinical decision support, a system that provides information to clinicians, staff, and patients to help inform decisions about a patient’s care, must keep patients informed and involved in reviewing results and data. However, much of our data operates in silos, and integrating AI into workflows requires new skills and training, such as writing effective prompts.
Audit trails and sharing best practices across the industry are also helpful in propelling mindful adoption.
AI Obstacles and Watch Outs
We are trying to be mindful of the lifecycle of adoption (think Gartner’s Hype Cycle).1 We know the stories in the news about the chatbots hallucinating.2 Issues around data quality and bias need to be taken into consideration and we need to establish audit trails and policy, and best practices need to be widely shared. Our historical interaction with technology is built on learning (new) skills, so we need to introduce this new training into our workflow, and we must learn how to write prompts to assist with our AI efforts.
AI should augment, not replace, the clinician or clinical trial staff. We need to use AI as a tool for efficient patient communication. There’s inherent distrust of technology; for example, many people want to know from whom they are getting their information. Until we can trust the ‘realness’ of AI, we will not arrive at the place of widespread adoption in our industry.
Machines are not inherently empathetic, so we must remember this vital ingredient in clinical trials – empathy. Remember, we are building technology solutions, and in the context of communicating complex messages, we cannot depend on AI to be the only end game.
To navigate the complexities of AI adoption in your clinical trial, contact us. We’re ready to continue the conversation when you are.
References:
- Gartner. Gartner Hype Cycle. https://www.gartner.com/en/research/methodologies/gartner-hype-cycle?utm_source=google&utm_medium=cpc&utm_campaign=GTR_NA_2022_GTR_CPC_SEM1_BRANDCAMPAIGNMQ&utm_adgroup=141653137818&utm_term=gartner%20hype%20cycles&ad=679713141030&matchtype=p&gad_source=1&gclid=Cj0KCQjwsuSzBhCLARIsAIcdLm7hTHTlywGGZVhu2A3fIWQLsrOuBOVQQK1gjHtx6CY6IzwLkNBu5i0aApCAEALw_wcB
- The New York Times. Chatbots May ‘Hallucinate’ More often Than Many Realize. https://www.nytimes.com/2023/11/06/technology/chatbots-hallucination-rates.html